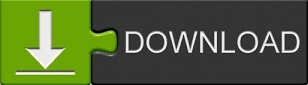
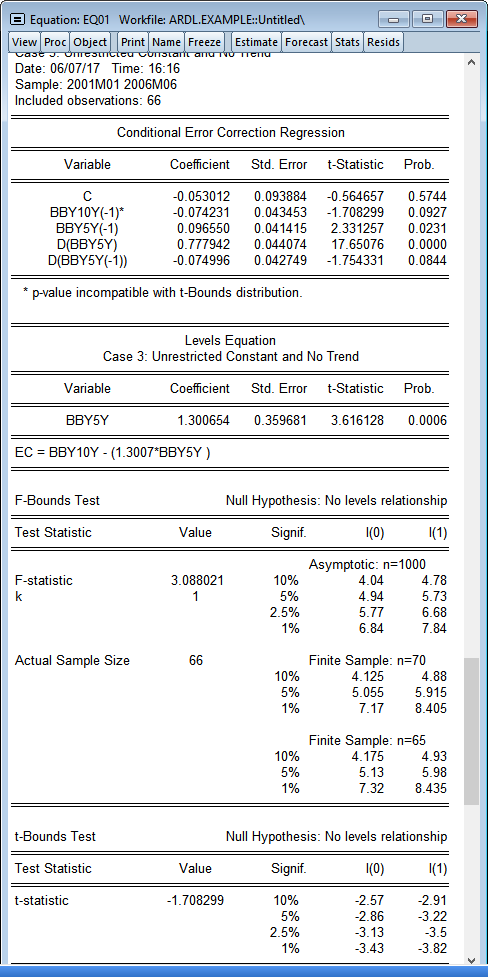
svar inflation unrate ffr, lags(1/6) aeq(A1) beq(B1)Įxactly identified model Log likelihood = -298.8751 The following code block loads the data, sets up the \(\bfA\) and \(\bfB\) matrices, estimates the model, then creates impulse responses and stores them to a file. Let’s revisit the three-variable VAR from the previous post, this time using svar. Stata’s svar command estimates structural VARs. However, different orderings embed different assumptions about the relationships among variables, and it may or may not be sensible to think that an impulse response will be robust to those differing assumptions. One might be tempted, as a sort of robustness check, to try multiple orderings to see whether impulse responses varied much when the ordering changed. The impulse responses one draws from the model are conditional on the ordering of the variables.
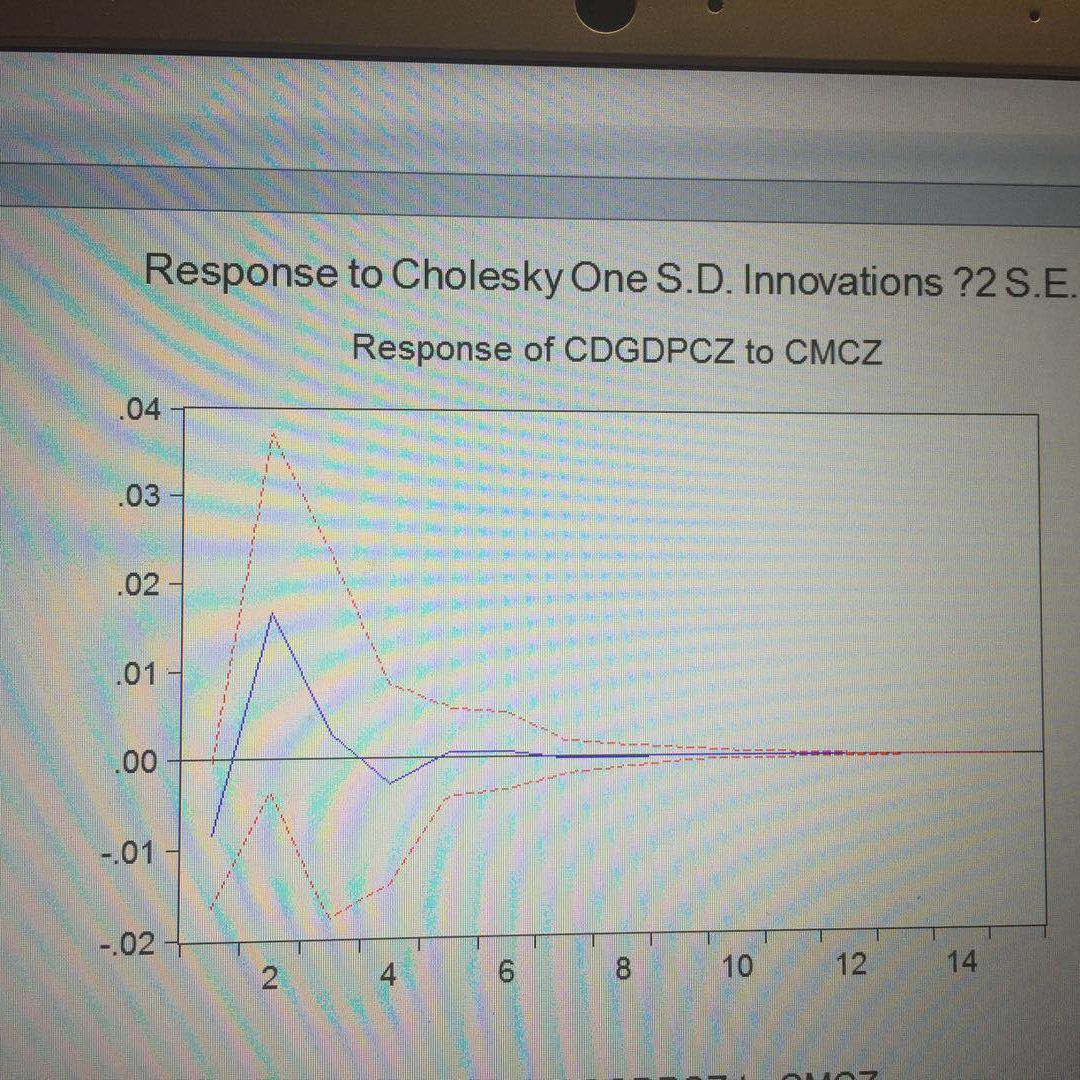
With Cholesky identification, order matters: permuting the variables in the VAR will permute the entries in \(\bfsig\), which in turn will generate different \(\bfB\) matrices. Different orderings reflect different assumptions about the underlying structure that the researcher is modeling. For example, if one believes that monetary policy only affects other variables with a lag, it is appropriate to place monetary instruments like the interest rate last in the ordering. This ordering may reflect some beliefs the researcher has about the various shocks. With the ordering (inflation, unemployment, interest rate), the shock to the inflation equation can affect all variables contemporaneously, but the shock to unemployment does not affect inflation contemporaneously, and the shock to the interest rate affects neither inflation nor unemployment contemporaneously. Suppose we have a VAR with three variables: inflation, the unemployment rate, and the interest rate. With this interpretation in mind, the causal ordering a researcher chooses reflects his or her beliefs about the relationships among variables in the VAR. Both of these methods may be thought of as imposing a causal ordering on the variables in the VAR: shocks to one equation contemporaneously affect variables below that equation but only affect variables above that equation with a lag. This identification scheme is often called “Cholesky” identification because the matrix \(\bfB\) can be recovered by taking a Cholesky decomposition of \(\bfsig\).Īn equivalent method of identification is to let \(\bfA\) be lower triangular and let \(\bfB=\bfI\). \def\bfsig hence, we can uniquely recover the structure from the reduced form.
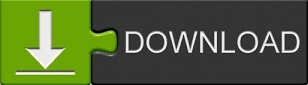